Applications For Data Analytics
Introduction to Data Analytics Applications
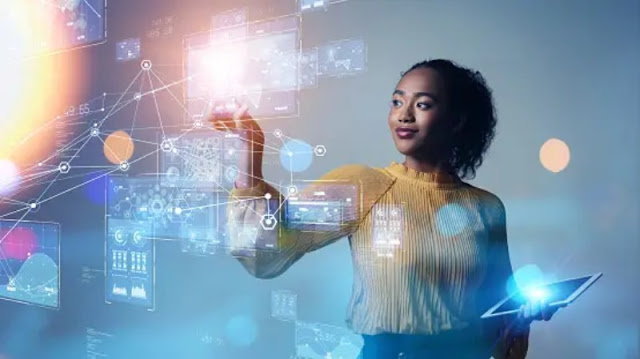
A. Definition and Significance of Data Analytics
1. Explanation of data analytics as the process of examining
data sets to draw conclusions
2. Importance in deriving actionable insights and informed
decision-making
3. Evolution of data analytics with technological
advancements
B. The Role of Data Analytics in Various Industries
1. Brief overview of how data analytics has transformed
industries
2. Mention sectors such as business, healthcare, finance,
manufacturing, etc.
3. Highlighting the potential for innovation, efficiency,
and competitiveness
C. Challenges and Opportunities in Data Analytics
Applications
1. Addressing issues like data quality, scalability, and
integration
2. Emphasizing the need for skilled data professionals
3. Noting the growing trend of AI and machine learning
integration
D. Outline of Subsequent Sections
1. Providing a roadmap for exploring various data analytics
applications
2. Teasing the range of sectors and use cases to be covered
in the following sections
B. The Role of Data Analytics in Various Industries
Business and Marketing
Customer Behavior Analysis: Understanding consumer
preferences, behavior, and purchasing patterns.
Sales and Revenue Optimization: Utilizing data to enhance
pricing strategies and identify upselling/cross-selling opportunities.
Marketing Campaign Effectiveness: Evaluating the impact of
marketing efforts through metrics like ROI and engagement.
Healthcare
Predictive Analytics for Patient Outcomes: Predicting
disease risks, treatment responses, and patient health trajectories.
Healthcare Resource Management: Optimizing resource
allocation, such as hospital beds and staff, based on demand patterns.
Fraud Detection and Prevention: Identifying fraudulent
claims and suspicious activities within the healthcare system.
Finance
Risk Assessment and Management: Using data to assess
creditworthiness, detect fraud, and manage financial risks.
Investment Analysis: Analyzing market trends and historical
data to make informed investment decisions.
Regulatory Compliance: Ensuring compliance with financial
regulations, such as anti-money laundering (AML) checks.
Manufacturing and Supply Chain
Demand Forecasting: Predicting product demand to optimize
inventory levels and supply chain operations.
Quality Control: Detecting defects and maintaining product
quality through data-driven monitoring.
Predictive Maintenance: Anticipating equipment maintenance
needs to reduce downtime and operational disruptions.
Social Media and Sentiment Analysis
Brand Reputation Monitoring: Analyzing social media sentiment
to manage brand perception and customer satisfaction.
Influencer Marketing Effectiveness: Identifying influencers
with the right audience and measuring campaign impact.
Environmental and Energy
Energy Consumption Analysis: Analyzing energy usage patterns
to suggest efficiency improvements and cost savings.
Environmental Impact Assessment: Measuring carbon emissions
and ecological impact to promote sustainability.
Transportation and Logistics
Route Optimization: Using data to find the most efficient
routes, reducing fuel costs and delivery times.
Vehicle Performance Monitoring: Analyzing vehicle data to
enhance fuel efficiency and schedule maintenance.
Education
Student Performance Analysis: Identifying struggling
students and tailoring interventions for better outcomes.
Institutional Resource Allocation: Allocating resources
effectively based on enrollment trends and budgets.
Others (Customize based on your needs)
Outline other industries where data analytics is crucial,
such as entertainment, agriculture, real estate, etc.
Conclusion
- Recap of the broad spectrum of industries benefiting from
data analytics.
- Mention how data-driven insights are reshaping business
strategies and improving outcomes.
- Transition to the next section highlighting challenges and
future tren
2. Course demand forecasting
In the context of the education sector, specifically higher
education institutions, course demand forecasting involves using data analytics
to predict different courses' popularity and enrollment patterns. This process
helps educational institutions make informed decisions about curriculum
planning, resource allocation, faculty assignments, and course offerings.
Here's a breakdown of the role and significance of course demand forecasting:
A. Understanding Course Demand Forecasting
Data Collection: Gathering historical enrollment data,
student demographics, academic trends, and external factors that might
influence course selection.
Data Analysis: Applying statistical and machine learning
techniques to analyze the collected data and identify patterns and correlations
between variables.
Prediction Models: Develop predictive models that use
historical data to forecast future course demand based on different variables,
such as time of year, academic calendar, student preferences, and external
events.
B. Importance and Benefits
Resource Allocation: Accurate forecasting helps institutions
allocate resources efficiently. For instance, they can determine how many
sections of a course to offer, how many faculty members are needed, and how to
distribute classrooms and teaching materials.
Curriculum Planning: Institutions can adjust their
curriculum offerings based on predicted demand. This prevents over-offering
courses with low enrollment and ensures the availability of popular classes.
Faculty Assignments: By knowing which courses will have
higher enrollment, institutions can assign faculty members effectively,
ensuring the right expertise for each class.
Optimal Class Sizes: Forecasting aids in maintaining
appropriate class sizes, which can impact the quality of education and student
experience.
Financial Planning: Accurate predictions allow for better
financial planning, preventing unnecessary spending on underutilized courses or
resources.
Student Satisfaction: Offering courses aligned with
students' preferences enhances their satisfaction and engagement with the
institution.
Efficient Scheduling: Forecasting helps create balanced
schedules that accommodate students' needs and preferences, reducing scheduling
conflicts.
C. Challenges
Data Quality: Accurate forecasting relies on reliable and
comprehensive historical data. Inaccuracies or missing data can lead to less
reliable predictions.
External Factors: External events, such as economic changes
or new industry trends, can impact course demand and might not be fully
accounted for in the data.
Complexity: The interplay of various factors makes
forecasting complex. Incorporating all relevant variables can be challenging.
D. Future Trends
Machine Learning Integration: Advanced machine learning
techniques can lead to more accurate predictions by capturing complex
relationships in the data.
Real-time Data: Utilizing real-time data and feedback loops
can enhance forecasting accuracy and adaptability.
Personalization: Customized course recommendations for
individual students based on their academic history and preferences could
become a norm.
Scenario Analysis: Develop models that can simulate the
impact of various scenarios (e.g., economic changes, industry shifts) on course
demand.
E. Conclusion
Course demand forecasting is pivotal in higher education
institutions' decision-making processes. By utilizing data analytics,
institutions can optimize their offerings, allocate resources effectively, and
enhance the overall student experience. As technology advances, the accuracy
and applicability of these forecasts are expected to improve, making them an
indispensable tool for educational institutions.